Techniques and Sampling Methods in Health Care
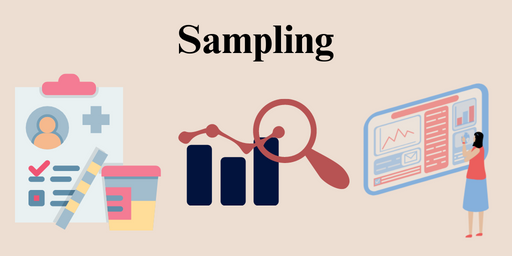
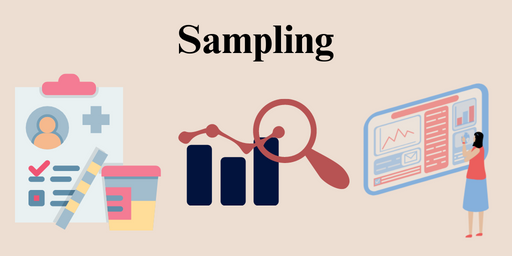
What is Sampling Method
Sampling is a process or way in which one selects a representative part of the population of interest to make valid inferences and generalizations. A sample is not only more feasible, economical, and practical than using the whole population; it is often more accurate.
A sample, in contrast to the greater number of cases in an entire population, decreases the likelihood of non-sampling errors such as measurement errors, non-response biases, and recording and coding errors. Most researchers think of sampling as important for accurately representing the population in descriptive terms, that is, external validity or generalization.
Sampling, however, is also concerned with the relationships found. Therefore, sampling errors or biases may threaten internal validity as well. Also, strictly speaking, samples are not “representative,” “unbiased,” or “fair” (Stuart, 1968).
Because the researcher
never knows the true population values, one cannot determine if any given
sample is truly representative of the population. It is the sampling process
that is representative, unbiased, or fair.
Types Random Sampling of Sampling
There are several types of sampling.
Simple random sampling
Simple random sampling is a procedure that may involve the use of a table of random numbers or the flip of a coin to determine who or what will be included in the sample. This approach, however, is often impractical and tedious and is infrequently used.
Systematic random sampling involves the use of a random start and then the selection of every kth case or incidence. This approach is more convenient than simple random sampling, but it can have variance estimation problems (Kish, 1965).
Systematic Random Sampling
A minimum of two systematic random samples with independent random starts is needed to estimate variance, unless one can assume a random distribution of the cases on the list used for sampling. Also, when using systematic random sampling, one must be careful that the list does not have some systematic order or periodicity. If so, systematic random sampling may lead to a seriously misrepresented sample or pattern.
For example, one might
inadvertently select all head nurses if the sampling interval mimicked the
sequencing of head nurses on the list. Or one might obtain blood samples only
when certain hormones are at their peaks if the sampling time interval mimicked
when the hormone peaked.
Stratified Random Sampling
Stratified sampling is another method of random sampling. It involves identifying one or more classification variables for sampling purposes. With stratified sampling, one randomly samples within each nonoverlapping stratum of the classification variables. For example, if sex is the classification variable, one randomly sample men and women separately.
Stratified sampling is intended to decrease sampling variability by increasing the homogeneity of the strata. For research purposes, it is best to select classification variables on the basis of their assumed association with the dependent variable, choosing those that are uncorrelated with each other.
Stratified sampling facilitates obtaining subgroup parameter estimates, may
increase the statistical efficiency of estimates if proportional allocation is
used, and may be more convenient if sampling lists are organized according to
the selected strata. However, stratified sampling also may be more costly and
complex and generally is applied to some, but not all, variables of interest.
Cluster Random Sampling
Cluster sampling is a fourth type of random sampling. Here the elements of interest for the study and the sampling units are not the same. The sampling unit or cluster is a convenient, practical, and economical grouping, such as practice sites, whereas the elements of interest for the study may be the individual patients obtained at the practice sites.
Thus, one randomly samples the clusters and takes all elements (or a relevant, random subset) within each cluster. In contrast to stratified sampling, where one samples from all strata of the classification variable, one samples only some clusters in cluster sampling. In contrast to desiring homogeneous strata, clusters should be as heterogeneous as possible.
To the extent that the clusters are. not
heterogeneous, one loses some precision, and the cluster sample is less
efficient than a simple random sample of the same size. At the extreme, if the
cluster is completely homogeneous, one achieves no gain from more than one case
per cluster.
Convenient Random Sampling
Finally, convenience samples, or non probability samples, are frequently used in nursing research. However, it is not possible to estimate sampling errors with such samples. Therefore, the validity of inferences drawn from nonprobability samples to the population remains unknown.
Furthermore,
whenever nonrandom selection is used, the potential for serious sample
selection biases exists. It is well-known that sample selection bias may
threaten internal as well as external validity. For this reason, sampling on
one's dependent variable should never be done.
Give your opinion if have any.